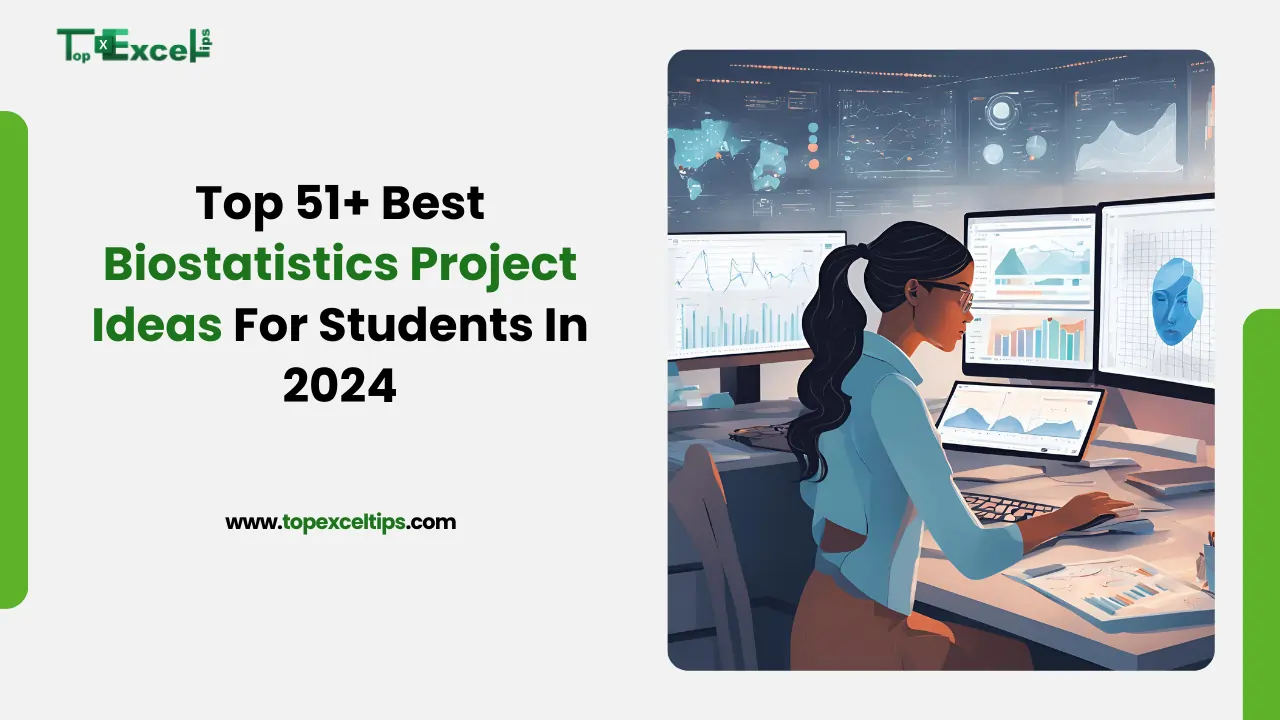
Biostatistics combines math, statistics, and biology to make sense of complex health data. It helps us understand disease patterns, predict future health trends, and make smart decisions that can improve public health and medical research.
Choosing the right project in biostatistics can really boost your learning and make a difference. Whether you’re interested in tracking diseases, studying clinical trials, or developing new methods, a good project can offer practical experience and valuable insights.
In this blog, we’ll explore a range of biostatistics project ideas that are both interesting and challenging. From analyzing public health data to creating new statistical tools, these ideas will help you use biostatistics to solve real-world problems. Whether you’re just starting or have some experience, you’ll find projects here that can help you make an impact in health and medicine.
Survey Results: Challenges in Choosing the Right Project Idea
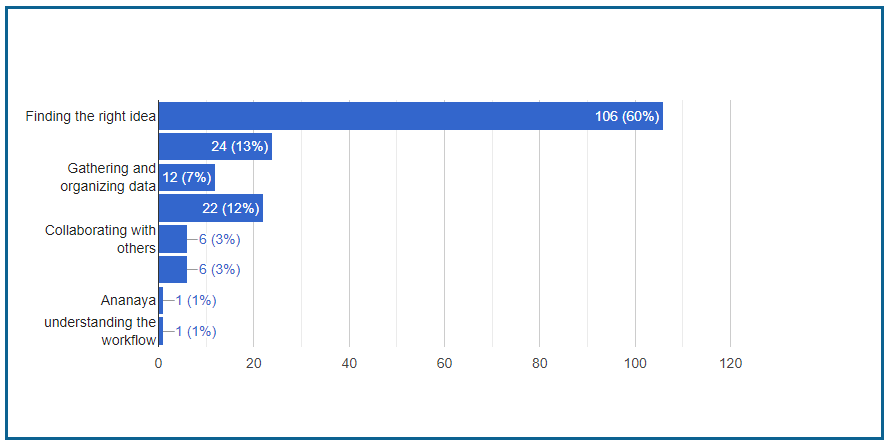
We recently polled 178 people and noticed that many struggled to select the best project idea. The majority of participants said they needed help choosing a project.
What Are Biostatistics Project Ideas and Why Are They Important?
Biostatistics project ideas involve using statistics to understand health and biological data. These projects help us answer important questions, solve real problems, and learn more about health and biology.
What Are Biostatistics Project Ideas?
These projects can include:
- Tracking Diseases: Studying data to see how diseases spread and predicting future outbreaks.
- Genetic Research: Looking at how genes affect the risk of diseases.
- Clinical Trials: Checking if new treatments work and are safe by analyzing trial data.
- Public Health Analysis: Examining health data to see how well health programs are working or finding trends.
Why Are They Important?
- Making a Difference: These projects provide insights that can lead to better health treatments and strategies. For example, analyzing clinical trials helps us know if a new drug is effective and safe.
- Learning by Doing: Working on these projects gives you practical experience with real data, which is important for learning and building skills.
- Discovering New Things: These projects can lead to discoveries and better ways to analyze data, helping us answer important health questions.
- Better Decisions: Good data analysis helps in making informed choices in healthcare and public policy. Projects that analyze health data can provide the information needed to create effective health solutions.
In short, biostatistics project ideas are essential for turning complex data into useful information. They help advance medical research, offer valuable learning experiences, and support better health decisions.
Top 53+ Biostatistics Project Ideas for Students in 2024
Here’s an expanded list of biostatistics project ideas with detailed sections to help you explore various aspects of the field. Each project idea includes a brief overview, objectives, potential data sources, and methods for analysis.
1. Disease Outbreak Analysis
- Overview: Study historical data to model and predict disease outbreaks.
- Objectives: Understand the patterns and factors contributing to disease spread.
- Data Sources: Historical epidemiological data, disease registries.
- Methods: Time series analysis, spatial modeling, predictive analytics.
2. Genetic Risk Factors
- Overview: Investigate how specific genetic variations influence disease risk.
- Objectives: Identify genetic markers associated with diseases like cancer or diabetes.
- Data Sources: Genome-wide association studies (GWAS) genetic databases.
- Methods: Association studies, logistic regression, pathway analysis.
3. Clinical Trial Evaluation
- Overview: Assess the effectiveness and safety of new treatments.
- Objectives: Determine how well new drugs or therapies work and their safety profile.
- Data Sources: Clinical trial data, electronic health records.
- Methods: Survival analysis, comparative effectiveness research, risk-benefit analysis.
4. Vaccination Impact
- Overview: Evaluate the effectiveness of vaccination programs.
- Objectives: Measure the reduction in disease incidence due to vaccinations.
- Data Sources: Vaccination records and public health reports.
- Methods: Pre-post analysis, cohort studies, regression analysis.
5. Environmental Health Analysis
- Overview: Study the impact of environmental factors on health outcomes.
- Objectives: Determine how environmental exposures like air pollution affect health.
- Data Sources: Environmental monitoring data, health surveys.
- Methods: Environmental epidemiology, spatial analysis, regression models.
6. Disease Progression Prediction
- Overview: Predict the progression of chronic diseases using patient data.
- Objectives: Forecast how diseases like diabetes or heart disease evolve.
- Data Sources: Longitudinal health records clinical data.
- Methods: Survival analysis, time series forecasting, machine learning models.
7. Health Interventions Assessment
- Overview: Analyze the success of public health interventions.
- Objectives: Evaluate how different health programs impact outcomes.
- Data Sources: Intervention records public health data.
- Methods: Pre-post comparisons, control group studies, cost-effectiveness analysis.
8. Survival Analysis
- Overview: Understand factors affecting patient survival rates.
- Objectives: Identify variables that influence survival for diseases like cancer.
- Data Sources: Cancer registries patient survival data.
- Methods: Kaplan-Meier curves, Cox proportional hazards model.
9. Biomarker Discovery
- Overview: Identify potential biomarkers for diseases.
- Objectives: Find biological indicators that signal disease presence or progression.
- Data Sources: High-throughput biological data, omics data.
- Methods: Biomarker identification techniques, statistical validation, machine learning.
10. Genomic Data Exploration
- Overview: Analyze GWAS data to find links between genetic variants and traits.
- Objectives: Discover genetic factors associated with various traits or diseases.
- Data Sources: GWAS datasets, genomic databases.
- Methods: Association analysis, polygenic risk scores, gene-environment interaction studies.
11. Drug Effectiveness Comparison
- Overview: Compare the effectiveness of different drugs.
- Objectives: Assess how different treatments perform in clinical settings.
- Data Sources: Clinical trial results and medication records.
- Methods: Comparative effectiveness analysis, meta-analysis, statistical testing.
12. Population Health Trends
- Overview: Identify trends and patterns in population health.
- Objectives: Understand how health indicators change over time across populations.
- Data Sources: National health surveys demographic data.
- Methods: Trend analysis, demographic analysis, statistical modeling.
13. Disease Spread Modeling
- Overview: Simulate how infectious diseases spread.
- Objectives: Model and predict the transmission dynamics of diseases.
- Data Sources: Epidemiological data, outbreak records.
- Methods: Mathematical modeling, simulation techniques, stochastic processes.
14. Longitudinal Data Study
- Overview: Study health changes over time within a cohort.
- Objectives: Track and analyze changes in health outcomes within a specific group.
- Data Sources: Longitudinal health surveys cohort studies.
- Methods: Longitudinal data analysis, growth curve modeling, mixed-effects models.
15. Cost-Effectiveness Analysis
- Overview: Evaluate the cost-effectiveness of health programs and interventions.
- Objectives: Determine the economic value of health interventions.
- Data Sources: Health program costs, outcome data.
- Methods: Cost-effectiveness ratios, incremental cost-effectiveness analysis, budget impact analysis.
16. Disease Risk Prediction
- Overview: Develop models to predict the likelihood of disease development.
- Objectives: Create predictive tools for assessing disease risk based on various factors.
- Data Sources: Health records, lifestyle surveys.
- Methods: Risk prediction models, logistic regression, and machine learning algorithms.
17. Quality of Life Research
- Overview: Study the impact of diseases and treatments on quality of life.
- Objectives: Assess how health conditions and interventions affect patients’ well-being.
- Data Sources: Quality of life surveys, patient-reported outcomes.
- Methods: Quality of life metrics, statistical analysis, comparative studies.
18. Clinical Decision Support
- Overview: Develop systems to assist clinicians with treatment decisions.
- Objectives: Enhance clinical decision-making using statistical models.
- Data Sources: Clinical data, decision support systems.
- Methods: Decision trees, risk assessment tools, machine learning.
19. Epidemiological Surveys
- Overview: Gather and analyze data on health behaviors and outcomes.
- Objectives: Understand health behaviors and their impact on outcomes.
- Data Sources: Survey data, health questionnaires.
- Methods: Survey design, data analysis, statistical inference.
20. Health Disparities Analysis
- Overview: Study differences in health outcomes among different groups.
- Objectives: Identify and understand health disparities and their causes.
- Data Sources: Public health data, demographic data.
- Methods: Disparity analysis, regression models, stratified analysis.
21. Big Data Statistical Methods
- Overview: Apply advanced statistical techniques to large health data sets.
- Objectives: Manage and analyze large-scale health data for insights.
- Data Sources: Big health data sets electronic health records.
- Methods: Big data analytics, high-performance computing, and advanced statistical methods.
22. Genetic Association Research
- Overview: Investigate links between genetic markers and diseases.
- Objectives: Discover genetic factors associated with specific traits or conditions.
- Data Sources: Genetic databases, GWAS data.
- Methods: Association studies, genetic linkage analysis, statistical genetics.
23. Patient Readmission Prediction
- Overview: Predict rates of patient readmission and identify contributing factors.
- Objectives: Develop models to forecast patient readmissions and improve care strategies.
- Data Sources: Hospital records, patient health data.
- Methods: Predictive modeling, risk factor analysis, machine learning.
24. Drug Interaction Analysis
- Overview: Study interactions between different drugs.
- Objectives: Understand how drug interactions affect patient health and treatment outcomes.
- Data Sources: Medication records clinical trial data.
- Methods: Interaction studies, statistical analysis, and drug interaction databases.
25. Healthcare Utilization Patterns
- Overview: Analyze patterns of healthcare use and their impact on outcomes.
- Objectives: Assess how healthcare services are used and their effects on health.
- Data Sources: Healthcare utilization data, patient records.
- Methods: Utilization analysis, statistical modeling, cost analysis.
26. Data-Driven Health Policy
- Overview: Use statistical analysis to recommend health policy improvements.
- Objectives: Inform policy decisions with data-driven insights.
- Data Sources: Health policy data, public health records.
- Methods: Policy analysis, data visualization, statistical inference.
27. Behavioral Health Analysis
- Overview: Examine mental health and behavioral conditions using data.
- Objectives: Identify trends and outcomes related to mental health.
- Data Sources: Behavioral health surveys, clinical data.
- Methods: Behavioral analysis, trend analysis, statistical modeling.
28. Machine Learning Applications
- Overview: Apply machine learning to biostatistical problems.
- Objectives: Use machine learning to predict disease risk or treatment outcomes.
- Data Sources: Health data sets, patient records.
- Methods: Machine learning algorithms, predictive modeling, data mining.
29. Diet and Health Studies
- Overview: Analyze the effects of different diets on health outcomes.
- Objectives: Understand how dietary patterns influence health.
- Data Sources: Dietary surveys and health records.
- Methods: Nutritional analysis, regression models, comparative studies.
30. Patient Satisfaction Analysis
- Overview: Study factors affecting patient satisfaction in healthcare settings.
- Objectives: Identify drivers of patient satisfaction and areas for improvement.
- Data Sources: Patient satisfaction surveys and healthcare feedback data.
- Methods: Satisfaction analysis, factor analysis, statistical testing.
31. Genetic Data Privacy
- Overview: Explore methods for protecting privacy in genetic research.
- Objectives: Develop strategies to safeguard genetic information.
- Data Sources: Genetic databases, privacy policies.
- Methods: Privacy-preserving techniques, data anonymization, and ethical analysis.
32. Environmental Health Risk
- Overview: Assess risks from environmental exposures.
- Objectives: Determine how environmental factors impact public health.
- Data Sources: Environmental exposure data, health outcome records.
- Methods: Risk assessment, exposure-response modeling, statistical analysis.
33. Comparative Effectiveness Research
- Overview: Compare the effectiveness of various health treatments.
- Objectives: Identify the most effective treatments based on real-world data.
- Data Sources: Treatment outcome data clinical trial results.
- Methods: Comparative effectiveness analysis, meta-analysis, statistical testing.
34. Health Data Visualization
- Overview: Create visual representations of health data.
- Objectives: Communicate insights and findings effectively through visuals.
- Data Sources: Health data sets, statistical analysis results.
- Methods: Data visualization tools, graphical representation, interactive dashboards.
35. Research on Rare Diseases
- Overview: Study rare diseases with limited data.
- Objectives: Explore characteristics and potential treatments for rare conditions.
- Data Sources: Rare disease registries, case studies.
- Methods: Statistical modeling, case series analysis, literature reviews.
36. Healthcare Cost Modeling
- Overview: Develop models to predict and analyze healthcare costs.
- Objectives: Understand and forecast healthcare expenses and their drivers.
- Data Sources: Cost data, healthcare utilization records.
- Methods: Cost modeling, economic analysis, regression models.
37. Chronic Disease Management
- Overview: Analyze data on managing chronic diseases.
- Objectives: Identify best practices and effective management strategies.
- Data Sources: Chronic disease management programs, patient data.
- Methods: Outcome analysis, intervention studies, statistical modeling.
38. Aging Population Health
- Overview: Study health outcomes and needs in aging populations.
- Objectives: Understand health issues and requirements for older adults.
- Data Sources: Aging population surveys, health records.
- Methods: Aging studies, demographic analysis, health trend analysis.
39. Health Economics Analysis
- Overview: Explore the economic aspects of health interventions.
- Objectives: Assess the cost and economic impact of health treatments.
- Data Sources: Economic data, health intervention records.
- Methods: Health economics modeling, cost-effectiveness analysis, economic evaluation.
40. Mental Health Risk Factors
- Overview: Identify risk factors associated with mental health disorders.
- Objectives: Discover factors that contribute to mental health issues.
- Data Sources: Mental health surveys clinical data.
- Methods: Risk factor analysis, statistical modeling, case-control studies.
41. Development of Biostatistical Tools
- Overview: Create new tools or software for biostatistical analysis.
- Objectives: Develop tools that enhance biostatistical research and data management.
- Data Sources: Biostatistical data sets software requirements.
- Methods: Tool development, software engineering, statistical analysis.
42. Health Campaign Evaluation
- Overview: Assess the effectiveness of public health campaigns.
- Objectives: Determine how well campaigns achieve their health goals.
- Data Sources: Campaign data, public health records.
- Methods: Evaluation techniques, effectiveness analysis, statistical testing.
43. Patient Records Analysis
- Overview: Study electronic health records to find patterns and trends.
- Objectives: Analyze patient records to identify trends and improve care.
- Data Sources: Electronic health records, patient data.
- Methods: Data mining, trend analysis, statistical modeling.
44. Personalized Medicine
- Overview: Use biostatistical methods to tailor treatment plans for individuals.
- Objectives: Develop personalized treatment strategies based on individual data.
- Data Sources: Patient data, treatment records.
- Methods: Personalized treatment modeling, statistical analysis, machine learning.
45. Healthcare Workforce Data
- Overview: Analyze data related to the healthcare workforce.
- Objectives: Understand staffing patterns and job satisfaction in healthcare.
- Data Sources: Workforce surveys, employment data.
- Methods: Workforce analysis, satisfaction surveys, statistical modeling.
46. Public Health Data Integration
- Overview: Combine data from various public health sources for comprehensive insights.
- Objectives: Integrate data to gain a holistic view of public health issues.
- Data Sources: Public health databases, research studies.
- Methods: Data integration techniques, comprehensive analysis, statistical modeling.
47. Patient Outcomes Analysis
- Overview: Study factors affecting patient outcomes across different settings.
- Objectives: Identify key factors that influence patient health outcomes.
- Data Sources: Patient outcome data, healthcare records.
- Methods: Outcomes analysis, factor analysis, regression modeling.
48. Vaccine Development Research
- Overview: Analyze data from vaccine trials to assess development and effectiveness.
- Objectives: Evaluate vaccine safety and efficacy through data analysis.
- Data Sources: Vaccine trial data, clinical studies.
- Methods: Efficacy analysis, safety assessment, statistical evaluation.
49. Bioinformatics Statistics
- Overview: Apply statistical methods to bioinformatics data.
- Objectives: Analyze genetic and protein data for biological insights.
- Data Sources: Bioinformatics databases, omics data.
- Methods: Statistical bioinformatics, data analysis, pattern recognition.
50. Surgical Procedure Outcomes
- Overview: Predict outcomes and risks associated with surgical procedures.
- Objectives: Assess the success and risks of various surgeries.
- Data Sources: Surgical data, patient records.
- Methods: Outcome prediction models, risk analysis, statistical evaluation.
51. Health Literacy Assessment
- Overview: Evaluate and improve health literacy across different population groups.
- Objectives: Understand and address gaps in health literacy.
- Data Sources: Health literacy surveys educational data.
- Methods: Literacy assessments, statistical analysis, educational research.
52. Ethics in Biostatistics
- Overview: Explore ethical considerations in data collection and analysis.
- Objectives: Address ethical issues in biostatistical research.
- Data Sources: Ethical guidelines, research data.
- Methods: Ethical analysis, case studies, policy review.
53. Remote Sensing for Public Health
- Overview: Use remote sensing data to study environmental factors affecting health.
- Objectives: Analyze how remote sensing data can inform public health research.
- Data Sources: Remote sensing data, environmental health records.
- Methods: Remote sensing analysis, environmental data integration, statistical modeling.
These project ideas are designed to help you dive deep into biostatistics, applying your knowledge to real-world problems and advancing the field in meaningful ways. Each project provides an opportunity to gain practical experience and contribute to important research.
Getting Started with Biostatistics: Key Resources and Requirements
To kick off and run a successful biostatistics project, you’ll need a mix of resources and tools. Here’s a straightforward guide to what you’ll need:
1. Understanding the Project Scope
- Clear Goals: Start by defining what you want to achieve with your project.
- Background Research: Dive into existing studies and data to get a good grasp of your topic.
2. Data Collection and Management
- Finding Data:
- Public Databases: Look for health-related databases and research archives (like NIH or WHO).
- Surveys: Create and distribute surveys to gather new data.
- Clinical Data: Use data from hospitals, clinics, or clinical trials if needed.
- Managing Data:
- Databases: Use tools like SQL or NoSQL for storing your data.
- Data Cleaning: Employ software like OpenRefine or Python’s Pandas library to clean your data.
3. Statistical Software and Tools
- Analysis Software:
- R: A free tool with a lot of packages for different kinds of statistical work.
- SAS: Great for handling large data and performing complex analyses.
- SPSS: Common in social sciences for statistical analysis.
- Stata: Useful for a range of data analysis tasks.
- Programming Languages:
- Python: Leverage libraries such as SciPy, NumPy, and Statsmodels.
- MATLAB: Ideal for detailed mathematical modeling.
4. Computational Resources
- Hardware:
- Computer: Make sure your computer can handle the processing needed for your analysis.
- Server Access: Consider using servers for more intensive data processing.
- Cloud Services:
- Cloud Platforms: AWS, Google Cloud, or Microsoft Azure can help with processing and storage.
- Data Storage: Use cloud solutions to manage large amounts of data.
5. Skills and Expertise
- Statistical Knowledge: Understand key statistical methods and data analysis techniques.
- Programming Skills: Be comfortable using tools like R or Python.
- Domain Knowledge: Know the field you’re working in, whether it’s epidemiology or genetics.
- Data Visualization: Learn to present your data clearly using tools like Tableau or Matplotlib.
6. Project Management
- Plan: Outline your project’s goals, timeline, and key milestones.
- Collaboration: Use tools like Trello or Asana to keep track of tasks and communicate with your team.
- Documentation: Keep thorough records of your methods and findings.
7. Ethics and Compliance
- Ethics Approval: Get approval from relevant ethics committees or review boards.
- Data Privacy: Follow regulations for protecting personal information, like GDPR or HIPAA.
8. Funding and Budget
- Funding: Look for grants or institutional support to finance your project.
- Budget: Manage your budget to cover expenses like software and data acquisition.
9. Publication and Dissemination
- Reports: Write detailed reports or research papers on your findings.
- Publication: Submit your work to journals or conferences.
- Presentations: Share your results through presentations at conferences or with stakeholders.
Having these elements in place will set you up for a successful biostatistics project and help you tackle your research effectively.
Final words
To sum up, starting a biostatistics project involves a few key steps. If you’re looking at the Top 51+ Best Biostatistics Project Ideas For Students In 2024, here’s what you need to do:
First, decide what you want to achieve with your project. Next, gather and manage your data using the right tools and sources. Make sure you choose suitable software and programming languages for your analysis.
Improve your skills in statistics and programming to better understand your data. Manage your project by planning your schedule, working well with your team, and keeping good records.
Follow ethical guidelines to protect personal information and consider finding funding to cover any costs. Finally, share your results by writing reports, submitting them to journals, and presenting your findings.
FAQs
What tools and software will I need?
You’ll need statistical software such as R, SAS, SPSS, Stata, or MATLAB. If you’re using Python, libraries like SciPy, NumPy, and Statsmodels are useful. You might also need data management tools and cloud services for handling large datasets.
How should I handle data for my project?
Collect data from reliable sources, such as public databases, surveys, or clinical trials. Use data management tools to store and clean your data. Follow ethical guidelines to protect personal information and ensure data privacy.
What should I include in my final report or presentation?
Your report or presentation should clearly summarize your project’s goals, methods, results, and conclusions. Highlight important findings and use visuals like graphs or charts to illustrate your data.